2022-07-28 アメリカ合衆国・マサチューセッツ工科大学(MIT)
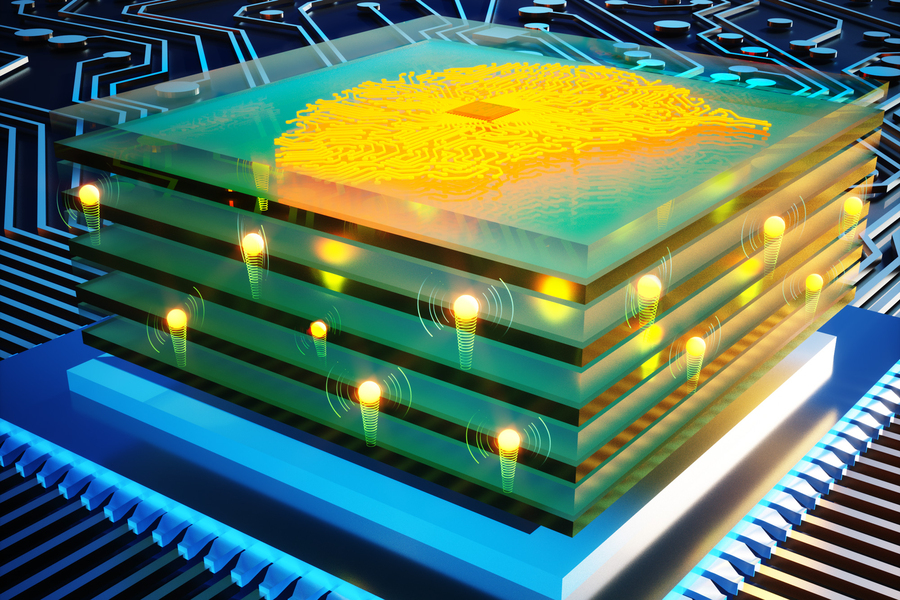
This illustration shows an analog deep learning processor powered by ultra-fast protonics.
Credit: Ella Maru Studio, Murat Onen
・ MIT が、アナログ深層学習(DL)アプリケーションに向けたプログラマブル抵抗器を開発し、その効果的な働きを実証。
・ 機械学習の能力強化に向けて複雑化するニューラルネットワーク(NN)モデルの訓練にかかる時間、エネルギー、コストは急増している。アナログ DL と呼ばれる新しい AI 分野は、より少ないエネルギーでの高速演算を可能にするもの。
・ アナログ DLでは、メモリでの演算実行と並列処理により、デジタルよりもエネルギー効率に優れる。
マトリクスサイズを拡張することで同時演算が可能となり、新しいタスクに追加的な時間がかからない。
・ リンケイ酸ガラス(PSG)を利用するため、抵抗器のエネルギー効率を飛躍的に向上させ、既存のシリコン製造技術によるナノスケールでのデバイス製造が可能に。商用コンピューティングハードウェアへの統合の可能性が期待でき、NN の訓練速度を大幅に向上させながらそのコストとエネルギーを低減するため、自動運転車や医療画像解析等用の DL モデルの開発を促進する。
・ プログラマブル抵抗器は、デジタルプロセッサではコア要素のトランジスタに相当する、アナログ DLの構成要素。ナノスケール薄の特殊なガラスフィルムデバイスで、デジタル NN のように演算を実行する。アナログの人工「ニューロン」と「シナプス」による NN では、画像認識や自然言語処理等の複雑なAI タスクが遂行できる。
・ 同抵抗器は、プロトンを伝導して電子を遮断する PSG 製の固体電解質が導電性の増減を調整することで作動する。PSG のナノポア表面がプロトン拡散の経路を提供し、強力な電場を利用してもプロトンを損傷せずにその動きを加速させ、ナノ秒の作動領域を達成。プロトンによる材料損傷も無いため、抵抗器は室温下で数 100 万回サイクルを作動する。
・ リチウムイオン電池等で見られる、インターカレーション反応のメモリデバイスへの応用は広く研究されている。今回実証したプロトンベースメモリデバイスの優れた切替え速度と持続性は、DL アルゴリズムを強化する新しいクラスのメモリデバイスの基礎を築くもの。
・ 次には、抵抗器の大量生産に向けた再設計や電圧の制限への対処を予定。本研究は、MIT-IBM Watson AI Lab が一部支援した。
URL: https://news.mit.edu/2022/analog-deep-learning-ai-computing-0728
<NEDO海外技術情報より>
関連情報
Science 掲載論文(アブストラクトのみ:全文は有料)
Nanosecond protonic programmable resistors for analog deep learning
URL: https://www.science.org/doi/10.1126/science.abp8064
Speed limit for solid-state nanoionics
The speed of biological information processing in neurons and synapses is limited by the aqueous medium through which weak action potentials of about 100 millivolts propagate over milliseconds. Above 1.23 volts, liquid water decomposes. Artificial solid-state neurons are not limited by such time and voltage constraints and can also be fabricated at the nanoscale, 1000 times smaller than their biological counterparts. Using complementary metal-oxide semiconductor–compatible materials, Onen et al. prototyped nanoscale protonic programmable resistors that can withstand high electric fields of around 10 megavolts per centimeter and which have energy-efficient modulation characteristics at room temperature. The proposed devices are 10,000 times faster than biological synapses and offer a promising direction for implementing various applications that can benefit from fast ionic motion. —YS
Abstract
Nanoscale ionic programmable resistors for analog deep learning are 1000 times smaller than biological cells, but it is not yet clear how much faster they can be relative to neurons and synapses. Scaling analyses of ionic transport and charge-transfer reaction rates point to operation in the nonlinear regime, where extreme electric fields are present within the solid electrolyte and its interfaces. In this work, we generated silicon-compatible nanoscale protonic programmable resistors with highly desirable characteristics under extreme electric fields. This operation regime enabled controlled shuttling and intercalation of protons in nanoseconds at room temperature in an energy-efficient manner. The devices showed symmetric, linear, and reversible modulation characteristics with many conductance states covering a 20× dynamic range. Thus, the space-time-energy performance of the all–solid-state artificial synapses can greatly exceed that of their biological counterparts.