2022-05-05 アメリカ合衆国・カリフォルニア工科大学(Caltech)
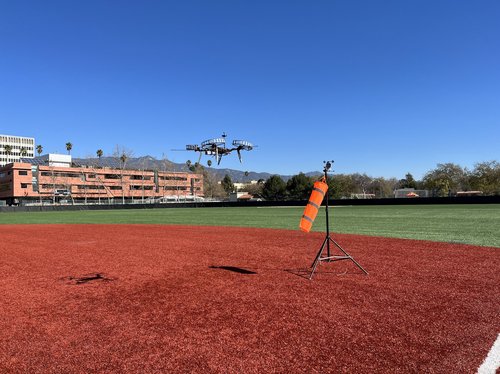
・ Caltech が、未知の天候や風況に無人航空機(ドローン)をリアルタイムに適応・飛行させる深層学習(DL)システム、「Neural-Fly」を開発。
・ 現在のドローンの飛行能力は、管理された無風の環境下や、遠隔操作等に制限されている。荷物の配送や交通事故による負傷者の空路での搬送等の日常的なタスクではドローンの自律的な飛行が必須となり、風況や天候にリアルタイムに適応する必要がある。
・ 流体力学モデルで適切な忠実度を達成し、それを各機体、風況や作動モードに合わせて調整することは難易度が高い。一方、機械学習(ML)では訓練に膨大な量のデータを要するが、古典物理学ベースの手法で達成した最先端の飛行性能には適合しない。さらに、深層ニューラルネットワーク(NN)のリアルタイムでの適応は、不可能ではないが大掛かりなタスクとなる。
・ 「Neural-Fly」は、ドローンに過去の経験を学習させて新しい条件にリアルタイムに適応させる、DL と適応制御を組みわせたアプローチ。移り変わる環境への効果的な対応に必要なパラメーターのみを更新するよう予め NN を訓練する、新しいメタ学習アルゴリズムを通じた分離的戦略を通じて前述の課題に対処する。
・ コンピューター制御した小型送風機 1,200 個超の 10 フィート四方のアレイから構成され、軽い突風から強風まであらゆる種類の風況をシミュレートする Real Weather Wind Tunnel により、八の字型の飛行経路で風速 12,1m/s(傘の使用が困難な雄風)の試験を実施。「Neural-Fly」は、クレジットカードサイズの Raspberry Pi4(約$20)で実行した。
・ 「Neural-Fly」によるドローンは、僅か 12 分間の飛行データの取得後に強風への対応を学習し、飛行性能を飛躍的に向上。同様な適応制御アルゴリズムを装備した最先端のドローンに比べ、飛行経路追跡のエラー率が約 2.5~4 倍低いことを確認した。NN によるドローン訓練時の 2 倍の風速に対応できたため、より厳しい天候の予測・汎化が可能。
・ 「Neural-Fly」は、DL でドローンの着陸位置と速度を追跡してドローンをスムーズに着陸させる「Neural-Lander」と、ドローン群に近接飛行を学習させる「Neural-Swarm」の過去に開発した両システムをベースとしている。
・ 本研究には、米国国防高等研究計画局(DARPA)とレイセオン(Raytheon)テクノロジーズが資金を提供した。
URL: https://www.caltech.edu/about/news/rapid-adaptation-of-deep-learning-teaches-drones-to-survive-any-weather
<NEDO海外技術情報より>
関連情報
Science Robotics 掲載論文(アブストラクトのみ:全文は有料)
Neural-Fly enables rapid learning for agile flight in strong winds
URL: https://www.science.org/doi/10.1126/scirobotics.abm6597
Abstract
Executing safe and precise flight maneuvers in dynamic high-speed winds is important for the ongoing commoditization of uninhabited aerial vehicles (UAVs). However, because the relationship between various wind conditions and its effect on aircraft maneuverability is not well understood, it is challenging to design effective robot controllers using traditional control design methods. We present Neural-Fly, a learning-based approach that allows rapid online adaptation by incorporating pretrained representations through deep learning. Neural-Fly builds on two key observations that aerodynamics in different wind conditions share a common representation and that the wind-specific part lies in a low-dimensional space. To that end, Neural-Fly uses a proposed learning algorithm, domain adversarially invariant meta-learning (DAIML), to learn the shared representation, only using 12 minutes of flight data. With the learned representation as a basis, Neural-Fly then uses a composite adaptation law to update a set of linear coefficients for mixing the basis elements. When evaluated under challenging wind conditions generated with the Caltech Real Weather Wind Tunnel, with wind speeds up to 43.6 kilometers/hour (12.1 meters/second), Neural-Fly achieves precise flight control with substantially smaller tracking error than stateof-the-art nonlinear and adaptive controllers. In addition to strong empirical performance, the exponential stability of Neural-Fly results in robustness guarantees. Last, our control design extrapolates to unseen wind conditions, is shown to be effective for outdoor flights with only onboard sensors, and can transfer across drones with minimal performance degradation.