2022-01-19 スイス連邦工科大学チューリッヒ校(ETHZurich)(チューリッヒ工科大学)
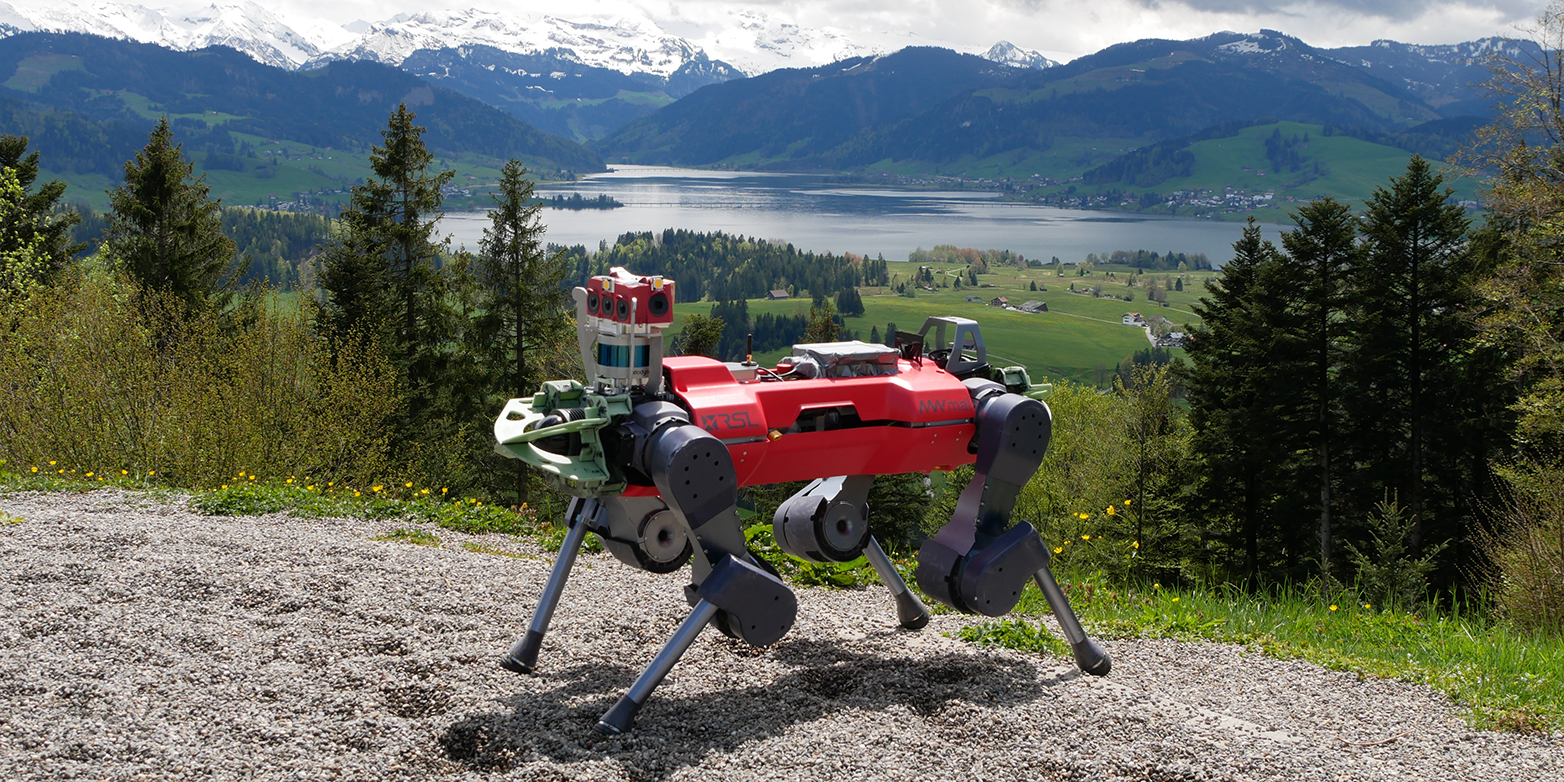
・ ETH Zurich が、ニューラルネットワーク(NN)による学習で視覚情報と触感覚を組み合わせ、四足歩行ロボット「ANYmal」による複雑な地形での歩行を制御するアプローチを開発。
・ 「ANYmal」は、同大学の Robotic Systems Lab が開発し、ETH のスピンオフである ANYbotics が商用化している。
・ 人間や動物は、自身の足や手の固有受容感覚と視覚から得た情報を自動につなぎ合わせ、視界が不明瞭であっても歩行困難な場所を通り抜けられる。
・ 四足ロボットでは、レーザーセンサーとカメラが捉える周囲環境情報が不完全なことが多いため移動可能な場所が制限される。例えば、実際には通り抜けられる背の高い草や浅い水たまり等は、対処不可能な障害物または部分的に不可視なものとして処理される。また、光、塵や霧による影響もある。
・ 新制御技術では、ロボットの足部が地面に接地する際の触感覚である固有受容感覚と周囲環境の視覚情報のつなぎ合わせを NN が学習し、ロボットにより高速で効率的に険しい地形を移動させる。
・ バーチャルトレーニングキャンプでの様々な障害物やエラー源との遭遇を通じ、ロボットが環境データを信頼または無視できるケース、また障害物を乗り越えるための理想的な方法を NN に学習させた。
・ その結果、ロボットは未経験かつ最も困難な自然地形での歩行方法を習得。周囲環境のセンサーデータが不明確な場合には、安全性を優先して固有受容感覚に判断を委ねる。
・ 新制御技術の実証では、ロボットが転倒や空足を踏むことなく、人間のハイキングよりも 4 分速い31 分間で 120m の高さの歩行を容易に完了。将来的には、危険性が高く人間や他のロボットには到達できない場所での利用を想定する。
・ 昨年9月開催のDARPA 地下チャレンジ(Subterranean Challenge)では、ETH Zurich はCERBERUSチームの一員として新制御技術の有効性を実証し、システム部門で優勝賞金の 2 百万ドルを獲得している。
URL: https://ethz.ch/en/news-and-events/eth-news/news/2022/01/how-robots-learn-to-hike.html
<NEDO海外技術情報より>
(関連情報)
Science Robotics 掲載論文(アブストラクトのみ:全文は有料)
Learning robust perceptive locomotion for quadrupedal robots in the wild
URL: https://www.science.org/doi/10.1126/scirobotics.abk2822
Abstract
Legged robots that can operate autonomously in remote and hazardous environments will greatly increase opportunities for exploration into underexplored areas. Exteroceptive perception is crucial for fast and energy-efficient locomotion: Perceiving the terrain before making contact with it enables planning and adaptation of the gait ahead of time to maintain speed and stability. However, using exteroceptive perception robustly for locomotion has remained a grand challenge in robotics. Snow, vegetation, and water visually appear as obstacles on which the robot cannot step or are missing altogether due to high reflectance. In addition, depth perception can degrade due to difficult lighting, dust, fog, reflective or transparent surfaces, sensor occlusion, and more. For this reason, the most robust and general solutions to legged locomotion to date rely solely on proprioception. This severely limits locomotion speed because the robot has to physically feel out the terrain before adapting its gait accordingly. Here, we present a robust and general solution to integrating exteroceptive and proprioceptive perception for legged locomotion. We leverage an attention-based recurrent encoder that integrates proprioceptive and exteroceptive input. The encoder is trained end to end and learns to seamlessly combine the different perception modalities without resorting to heuristics. The result is a legged locomotion controller with high robustness and speed. The controller was tested in a variety of challenging natural and urban environments over multiple seasons and completed an hour-long hike in the Alps in the time recommended for human hikers.